
In April 2018, the US Food and Drug Administration (FDA) made a momentous decision. The agency’s approval of IDx-DR, a diagnostic system developed by Iowa-based IDx Technologies for diabetic retinopathy, wasn’t a revolutionary move on the face of it, but nevertheless marked an important inflection point in the delivery of modern healthcare.
So why was the FDA’s decision to award marketing clearance to IDx-DR so significant? As is increasingly the case in medical technology, the answer lies with artificial intelligence (AI). The IDx-DR software is driven by AI, and it’s the first system approved to autonomously provide diagnostic assessments without the supervision of an expert clinician.
The system involves capturing images of a patient’s eye with a retinal camera – in this case the Topcon NW400 – that can be operated by any non-specialist staff member with a little training. The images are then uploaded to a cloud server for analysis by the IDx-DR software. Within a few minutes, the algorithm can produce a diagnostic interpretation for diabetic retinopathy and an associated report. The software is programmed to produce one of two results – either negative for more than mild diabetic retinopathy, in which case a re-screening in 12 months is recommended, or positive, for more than mild diabetic retinopathy, which generates a recommendation for a referral to an eye care specialist.
Removing the need for a dedicated specialist brings a cascade of benefits for patients and healthcare providers. The ability for a reliable test to be carried out in a primary healthcare setting could dramatically improve screening rates for diabetic retinopathy, as more patients are able to access a quick check-up during routine appointments. This increases the chance that clinics will be able to diagnose diabetic retinopathy earlier, which means that the vision loss associated with the condition could more often be prevented.
“Autonomous AI systems have massive potential to improve healthcare productivity, lower healthcare costs, and improve accessibility and quality,” said IDx founder and president Dr Michael Abramoff last year. “As the first of its kind to be authorized for commercialization, IDx-DR provides a roadmap for the safe and responsible use of AI in medicine.”
Diagnosis: AI is starting to outperform doctors
So while IDx-DR has huge clinical potential as an enabler of quicker and more accessible diagnosis for diabetes’s most feared complication, it’s not necessarily the technology itself that represents the major milestone. Rather, the roadmap that Abramoff mentioned is the true revolution; as machine learning algorithms continue to evolve and deepen, the med tech industry – and, increasingly, regulators – are opening up to the exciting and possibly intimidating prospect that smart software might be able to take over some tasks that previously required expert human input.
How well do you really know your competitors?
Access the most comprehensive Company Profiles on the market, powered by GlobalData. Save hours of research. Gain competitive edge.
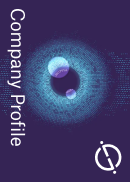
Thank you!
Your download email will arrive shortly
Not ready to buy yet? Download a free sample
We are confident about the unique quality of our Company Profiles. However, we want you to make the most beneficial decision for your business, so we offer a free sample that you can download by submitting the below form
By GlobalDataThere is considerable debate about the capacity for AI to dramatically change the delivery of healthcare, and where its limits might lie. Caution is key in a highly regulated industry, and in areas such as drug discovery or treatment guidance, the jury is still out, as IBM Watson for Oncology’s misadventures in cancer treatment recommendations have demonstrated.
But diagnostics, with its emphasis on identifying subtle patterns that can be hard to detect with the human eye, makes for an ideal starting point. Already, the reliability of many new AI-driven diagnostic systems is striking. The FDA’s approval of IDx-DR was chiefly based on a clinical study of retinal images from 900 diabetic patients at ten primary care sites, during which the system was able to detect more than mild diabetic retinopathy with an impressive 87.4% accuracy.
In many cases, machine learning algorithms have now been shown to equal or outperform their expert human counterparts in diagnostic accuracy. Last year, a study published in the Annals of Oncology journal by an international team of researchers found that a deep learning convolutional neural network (CNN) was superior to a group of 58 dermatologists at diagnosing skin cancer, catching 95% of melanomas compared to the human specialists’ 86.6%.
“The CNN missed fewer melanomas, meaning it had a higher sensitivity than the dermatologists, and it misdiagnosed fewer benign moles as malignant melanoma, which means it had a higher specificity; this would result in less unnecessary surgery,” said the study’s first author, Professor Holger Haenssle of the University of Heidelberg. “When dermatologists received more clinical information and images at level II, their diagnostic performance improved. However, the CNN, which was still working solely from the dermoscopic images with no additional clinical information, continued to outperform the physicians’ diagnostic abilities.”
Is doctorless diagnosis on the horizon?
With AI-driven diagnostics becoming ever more impressive, there’s a somewhat uncomfortable question hanging in the air: is diagnostics destined to become a realm of the machines, with human input surplus to requirements?
It’s certainly an open question, and one that draws a wide range of opinions. In a 2017 interview with The New Yorker, University of Toronto computer scientist Dr Geoffrey Hinton was blunt about the impact of AI on the field of radiology.
“I think that if you work as a radiologist you are like Wile E. Coyote in the cartoon,” Hinton said. “You’re already over the edge of the cliff, but you haven’t yet looked down. There’s no ground underneath. It’s just completely obvious that in five years deep learning is going to do better than radiologists.”
In an editorial for the British Medical Journal, Professor Jörg Goldhahn, deputy head of ETH Zurich’s Institute of Translational Medicine, similarly argued that the challenge of making machines universally superior to human doctors is “technical rather than fundamental because of the near-unlimited capacity for data processing and subsequent learning and self-correction…These systems continually integrate new knowledge and perfect themselves with [a] speed that humans cannot match.”
Certainly with the latest advancements in deep learning and neural networks, the most sophisticated AIs are effectively mimicking the learning process of the human brain, but with all the advantages of inexhaustible memory and none of the limits of human systems or complacency. After all, when a human doctor makes an error, it might be months or years before it becomes clear, and in many cases the diagnosing clinician would never become aware of their mistake.
Care at the centre: adapting to the rise of AI
With machine learning operating on another level to humans in terms of raw computational power, where does that leave doctors in the diagnostic field? In reality, while AI will continue to evolve and will certainly play an increasingly prominent role in tasks such as visual analysis, it’s difficult to envision a time when human physicians might be considered even close to obsolete in diagnostics.
While validated AI algorithms may be able to take over routine diagnosis of non-serious illnesses in the future, the human factor in the delivery of care can’t be overstated. Very few people, for example, would prefer to receive a cancer diagnosis via an impersonal screen readout rather than a lengthy consultation with a sympathetic human expert who can immediately answer their questions and discuss their options. Even in pattern recognition, machine learning’s diagnostic strong suit, humans have an edge of extra context about the patient’s background and lifestyle, stress levels, recent activities and so on.
The majority of these human advantages come from interpersonal communication. The rise of AI is unlikely to see doctors trained as supportive technicians, as some observers fear, but rather as expert communicators and empathisers. Scripps Research Translational Institute founder Dr Eric Topol – a cardiologist, geneticist and digital medicine researcher – has argued for years that the development of medical AI offers the opportunity to address the imbalance in physicians’ workloads and train a new generation of medical professionals with human factors at the forefront.
“We need the highest emotional intelligence, the best communicators, the people with the most compassion and empathy for others,” Topol said in an October interview with Aviva Investors. We need to adjust, as the ‘brainiac’ premium is going to be reduced when you have all this data-processing capability and AI that knows the literature and achieves a higher accuracy of diagnoses with fewer errors. It’s nice to have great minds, but we need to put an even higher priority on the humane elements, the essence of medicine, which is a connection between patients and their doctors.”
Medicine’s AI story is just beginning, and at this stage it’s natural that some commenters will frame it in oppositional ‘man vs machine’ terms. It’s certainly true that there is more work to be done to regulate the ethics and dynamics of machine learning in diagnostics, address potential bias in training data-sets, and set a framework that allows algorithms to play a role without increasing patient risk or scrubbing the humanity out of healthcare.
But the reality is that by taking over the time-consuming tasks that machines can do better than humans, AI has the potential to forge a true partnership with healthcare professionals, freeing them from certain jobs – from visual analysis in diagnostics to the mundane administration and reporting that takes up a disproportionate amount of their time – and allowing them to focus on connecting with patients. In medicine as much as any other sector, the adage holds true that two heads are better than one, even when one is organic and the other is a synthetic neural network trained on millions of data points.