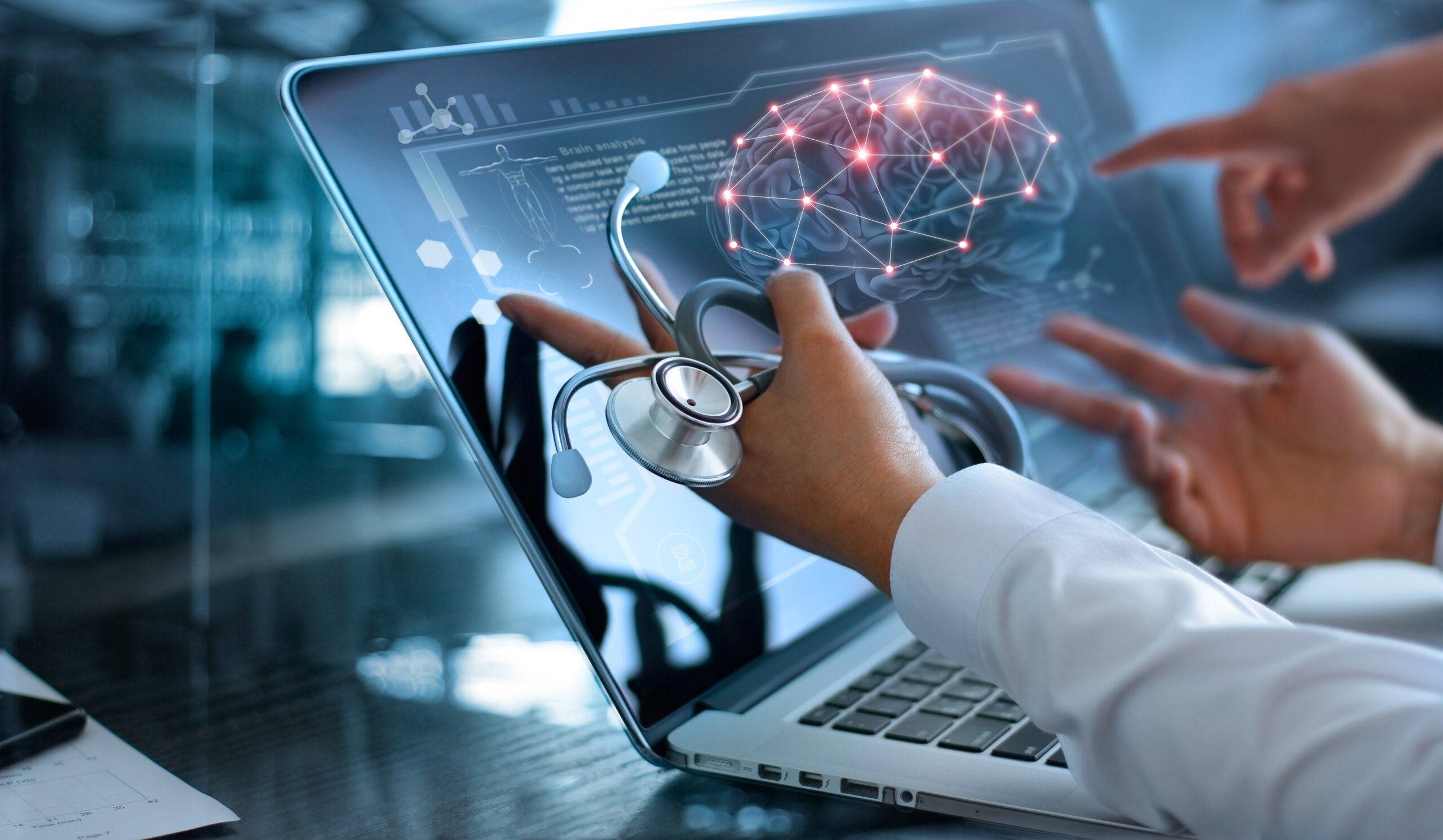
In 2022, 44% of countries responding to a World Health Organisation (WHO) survey reported they had experienced one or more disruptions to mental health care, including prevention and promotion programmes, diagnosis, treatment and life-saving emergency care.
Deepening value and commitment to mental health understanding, reshaping environments and strengthening mental health care are critical paths to transformation, the WHO states in its 2022 World Mental Health Report.
With democratising access to accurate and efficient mental health care a core issue in our global healthcare system, new tools and technologies are emerging to support clinicians and improve patient outcomes. Awareness of brain scanning and imaging technologies that use artificial intelligence (AI) in mental health understanding and diagnostics is particularly growing. “Brain imaging plays a critical role in mental health diagnosis,” says Bin He, professor of biomedical engineering at Carnegie Mellon University. As the role of the advancing tech in mental health progresses, research and development in the field is increasing and healthcare companies are launching new assistive tools.
The introduction of AI-led brain scanning tech
Limitations in traditional analytical approaches, namely a lack of focus on individual-level decision-making and the part different brain networks play in explaining brain disorders, led to the emergence of AI-led brain solutions, specifically the use of machine learning (ML) to understand mental disorders. Rather than examining brain regions independently, ML models look for undiscovered holistic patterns from data using advanced knowledge from applied statistics and mathematical optimisation techniques.
ML can generate individual-level diagnostic and prognostic decisions. “Standard machine learning (SML) models gained a varying degree of success, and the expert-led feature extraction and selection step is almost a prerequisite for its well-functioning,” says Md Mahfuzur Rahman, first author of a recent Georgia State University study and a doctoral student in computer science.
How well do you really know your competitors?
Access the most comprehensive Company Profiles on the market, powered by GlobalData. Save hours of research. Gain competitive edge.
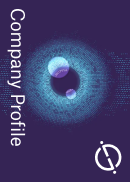
Thank you!
Your download email will arrive shortly
Not ready to buy yet? Download a free sample
We are confident about the unique quality of our Company Profiles. However, we want you to make the most beneficial decision for your business, so we offer a free sample that you can download by submitting the below form
By GlobalDataHowever, when trained on raw data, SML models did not perform well. “We need to go beyond existing knowledge, and learning from the raw data is essential for further advancement in mental health analysis,” adds Rahman.
Developing a greater understanding of the data obtained is key. “Direct learning from the data may reveal undiscovered and valuable patterns within the data and bring translational value to clinical practices,” says Rahman. The approach may also accelerate diagnostic and prognostic decision-making processes, eventually leading to personalised treatment plans.
In contrast to SML models, deep learning (DL) has been “very popular” because it does not require prior feature selection or intermediate intervention, Rahman notes. It can learn automatically from the raw data and find discriminative and potentially useful clinical features.
AI-centred research
A growing body of research into AI-based brain imaging technology is advancing the scientific community’s understanding of how it can assist in mental health care.
New research by Carnegie Mellon University’s College of Engineering has developed brain imaging technology based on AI. The discovery adds to the existing body of tools that have been, to date, used to study brain activity, such as MRI, electroencephalography (EEG) and magnetoencephalography.
The study, headed up by professor of biomedical engineering Bin He, found the AI-led technology was capable of mapping out rapidly changing electrical activity in the brain and suggests it can deliver this information with high speed, resolution and at low cost.
“We found that AI-based dynamic brain imaging techniques can provide superior imaging performance, including precision and specificity, than conventional imaging algorithms,” says He.
New research by Georgia State University’s (GSU) TReNDS Center explores the potential of an advanced computer system to help in the early diagnosis of conditions, including schizophrenia. Researchers examined brain imaging data to explore distinctive patterns linked to mental health conditions. Using functional magnetic resonance imaging (fMRI) and building AI models to interpret the outcomes, the researchers could measure dynamic brain activity by assessing tiny blood flow changes.
King’s College London (KCL) has researched an AI-led supercomputer designed to develop deep learning models to better understand human brains. KCL strives to advance and accelerate health research in several fields, including medical imaging, genetics and medication development. Developing its Synthetic Brain Project, KCL seeks to produce deep learning models and create 3D MRI imagery of human brains to build knowledge across ages, genders and disorders.
Commercialising AI-based tools
Increasing understanding, interest and discovery of the possibilities for AI-led brain scanning technologies in mental health diagnostics is seeing tech companies develop and launch devices and tools.
Australian health tech company Annalise has released its AI-enabled software-as-a-medical device (SaMD) decision-support solution for non-contrast CT brain studies for clinical use. The assistive clinical tool aims to ease the burden on radiology services and help improve diagnostic accuracy and patient health outcomes.
Polish AI solutions developer Brain Scan has designed its AI-based technology to help overcome the delays hospitals face in receiving imaging interpretations and improve radiology workflow to reduce under-reporting and patient prioritisation.
South Korean digital mental health platform iMediSync has launched an AI-based brain-scanning device. The remote-care mental health platform tool is a helmet-like device that measures brainwaves. Developed to offer efficient and convenient insights into brain activity, the device establishes the degree of activation in various parts of the brain, including frontal and temporal lobes, allowing for brainwave performances to be made with patients in the same age demographic.
Developing our understanding of the brain
The scientific team at Carnegie Mellon University is building on its current research. “We are further refining the technology to make it easier to use and test its applicability on general neuroscience research and application to mental health diagnosis,”says He.
Recent scientific findings have indicated that DL performs well for mental disorder diagnosis and prognosis tasks, Rahman states.“However, DL models are black boxes, and their learning mechanism is still not fully understood,” says Rahman. “We must go beyond diagnosis and understand what the model has learned from the data.”
Referring to recent mental disorder studies, Rahman highlights these have paid “enormous attention” to a growing subfield in AI, traditionally called Explainable AI (XAI), to uncover the knowledge the ML/DL models have learned from the brain data. While this field has achieved initial success over multiple disorders and is aligned with existing literature, Rahman explains these findings, in their current forms, are insufficient for individualised treatments in everyday clinical practices.
“However, the existing best models can play essential roles as supportive tools for individualised diagnosis and prognosis, validating the decisions made by human experts,” says Rahman.
Future potential in mental health care
AI-led brain scanning technology offers a novel means to allow better brain imaging of mental processes and mental diseases, He says. “It may make dynamic brain imaging a routine diagnostic procedure to diagnose various mental disorders and guide neuromodulation treatment of mental diseases,” He adds.
However, learning more about how AI models interpret and use data from brain scanning technology is vital to ensure comprehensive understanding and accurate use. “Importantly, there is a massive chance of clinical misuse with potential adverse outcomes if the model is prematurely designed, trained, tested, and deployed,” Rahman states.
Therefore, identifying AI-led tech’s role in helping better understand and diagnose a variety of mental health conditions is crucial. “Further research should be conducted to test its direct application to various disorders affecting mental health,” He adds.
While AI models, such as those generated in KCL’s recent research, were designed to diagnose neurological conditions, the technology may have the capability to anticipate potential future brain disease and growth, identifying and advancing preventative therapy options. “As the field of interpretability in neuroimaging is flourishing rapidly, we believe AI-led solutions will soon be efficient enough to encompass the entire trajectory of diagnosis, prognosis, and treatments,” Rahman concludes.