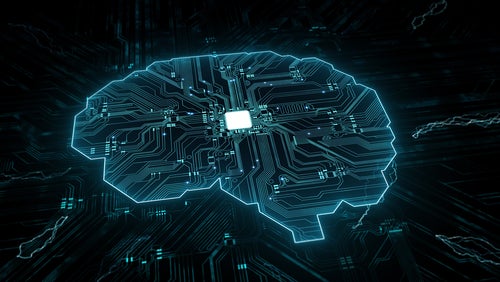
Autism is a complex condition that can affect an individual’s communication skills, interests and behaviour. It can present very differently from patient to patient, meaning diagnosis isn’t always straightforward and some patients aren’t diagnosed until adulthood.
Symptoms tend to present during the first year or so of a child’s life, and early diagnosis can be hugely beneficial to their wellbeing as they grow up. Non-White children, females and children from rural areas or poorer socioeconomic backgrounds are the most likely to struggle to receive a diagnosis, largely due to a lack of diversity in autism research.
In June, the US Food and Drug Administration (FDA) approved an artificial intelligence (AI) platform to help diagnose autism. Cognoa’s Canvas Dx is a machine learning mobile app designed to help healthcare providers diagnose autism in children aged 18 months through to five years old who exhibit potential symptoms of the disorder. Established by the ‘bad boy of autism research’ Dr Dennis Wall, the company aims to help facilitate earlier diagnoses for autistic children.
The device is not designed to replace a specialist diagnosis, but to assist physicians in providing their diagnosis earlier and more efficiently.
It consists of three components: a mobile app for caregivers and parents to answer questions about behaviour and upload videos of their child; a video analysis portal that allows certified specialists to view and analyse the videos; and a healthcare provider portal where clinicians can enter answers to pre-loaded questions about behaviour, track the information provided by parents or caregivers and review the results.
The algorithm will then give a diagnosis or say that no result can be generated if the information given is insufficient.
How well do you really know your competitors?
Access the most comprehensive Company Profiles on the market, powered by GlobalData. Save hours of research. Gain competitive edge.
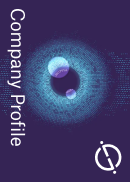
Thank you!
Your download email will arrive shortly
Not ready to buy yet? Download a free sample
We are confident about the unique quality of our Company Profiles. However, we want you to make the most beneficial decision for your business, so we offer a free sample that you can download by submitting the below form
By GlobalDataMedical AI has been historically criticised for encoding demographic biases. A high-profile 2019 study found that a prominent AI software used in US hospitals to determine which patients received access to high-risk healthcare management programmes routinely prioritised healthier White patients over less healthy Black patients.
More recently, a report published by the Center for Applied Artificial Intelligence at the University of Chicago Booth School of Business found that several algorithms used to inform healthcare delivery across the US were reinforcing racial and economic biases.
Conversely, Cognoa maintains that its platform can help make early diagnoses more accessible to all children, “regardless of gender, ethnicity, race, zip code, or socioeconomic background”. Medical Technology speaks to Cognoa chief medical officer Dr Sharief Taraman to find out more about how Canvas Dx works and the importance of unbiased AI.
Chloe Kent: How does AI end up biased in the first place?
Sharief Taraman: We already have a tonne of biases baked into the healthcare system, but now that we have AI coming in as a new tool it’s magnifying things that were already there.
We have to be very intentional and thoughtful about we apply AI in medicine so that we don’t unintentionally magnify or exacerbate existing biases in the healthcare system.
AI is only as good as the data you train it on and the collaboration between data scientists, clinicians and other important stakeholders like patient advocacy groups in developing it.
If we’re very intentional about making sure we include all of those folks, we do it in a way that actually removes the biases and gets rid of them.
CK: So rather than encoding biases, AI could actually help to eliminate them?
ST: I think we need to be thoughtful about how we apply AI and make sure that we have diverse training datasets. When we apply the AI we need to continue to monitor it and use it not as a replacement for physicians but instead as something that adjuncts their abilities.
If you do that, then you’re in a good place. You have enough safeguards in there that I think the equities should be good and the disparities should be minimised and not amplified.
We also need to make sure the technology is accessible. For example, if you have an iOS-only device you’re losing the huge demographic of people who can’t afford or do not have one.
One of the things that we were very intentional about was creating a socially responsible AI charter for our organisation.
CK: What is the content of your socially responsible AI charter?
ST: The data that you train on has to be ethnically, racially and socioeconomically diverse. We know in autism, in the existing infrastructure, the research tools that are being used to diagnose kids were normalised and trained on white males.
This means that if you’re anything other than a white male you’re more likely to be misdiagnosed, never diagnosed or diagnosed on average much later than a white male.
Although we used some of that information to help us understand how we were going to build the AI, we tried to collect data that was actually diverse when we collected data of our own.
We wanted to make sure that we recruited a test group that was diverse, to see if there was a difference in the way the device performs in different groups: if you have a lower socioeconomic status, if you have a higher socioeconomic status, if you’re in this geographic location versus that, if you’re black or Hispanic or white. What we found is that there were no differences in those groups.
One of the other challenges that you can get is this drift in the algorithm. As people or devices or healthcare changes, does the algorithm still work the way it did five or ten years ago? One of the things that we’re committed to is that we need to continue to monitor the product and make sure that it still works the way that we thought it worked, that it’s not missing or misdiagnosing kids.
CK: How else can algorithm developers ensure that what they’re building is as unbiased as possible?
ST: For medical AI specifically, I think there are two really big tenets that you have to deploy. You can’t just have tech folks doing this in a silo, they really do need to involve clinicians, but we also need to train physicians on how to understand AI.
As a physician, if I don’t understand the informatics or the artificial intelligence part of this, I can’t have a meaningful conversation with a data scientist. Just like we teach doctors how to use a stethoscope, we need to teach them what AI is, what it can and can’t do and how to interpret it.
CK: How can AI interact with demographic-related disparities in autism care?
ST: One of the challenges it that autism is this very heterogeneous condition. I might have a kid who comes into my office who is crawling all over me and then the next kid I see is hiding under the table. They both have autism, but the way that looks is very different.
It’s really about trying to pick out the challenges for each of the individual patients, regardless of their sex, race, ethnicity, etc.
The beautiful thing about AI is that AI is really good at doing that. The existing tools that I use as a specialist, they’re what we call linear. I don’t want to be too crass but it’s kind of like a Cosmo quiz, ‘does my boyfriend or girlfriend love me?’, whatever.
You answer the questions and then you get a score at the end and you’re like ‘oh, I hit threshold, I have autism’, but that’s actually a really rudimentary way of thinking about it and it has this risk for false classification.
With AI, if I want to look at 64 behavioural features, I can look at every feature in relation to every other feature. It’s not linear, it doesn’t hit this threshold for diagnosis, it’s saying “this child’s eye contact is this way, their socialisation is that way, let me look at how these two are related”.
Then we can compare those factors to a third variable. And the computer can do that with all the different factors all day long to generate this high dimensional picture of the patient’s presentation.
CK: Would you ever consider expanding your tool to any other indications, like diagnosing adults with autism or other neurodiverse conditions like attention deficit hyperactive disorder (ADHD)?
ST: In our roadmap we are more on the paediatric side right now, really focusing on that early developmental window, but we do have the ability to expand upwards by age into other conditions.
For every child that we evaluated with autism, we also had a number of learnings about ADHD, anxiety, obsessive-compulsive disorder, oppositional defiant disorder, so those things are part of our pipeline development.
The other thing that we’re really focusing on is that – not only was there no FDA evaluated tool to diagnose autism – the only FDA-approved treatments for autism right now are atypical antipsychotics. You can imagine what the side effect profile looks like in a three or four-year-old, it’s not something that most clinicians want to use.
One of the things that came out of Dr Wall’s lab is an augmented reality (AR) and AI based solution to really help parents and their children work on socialisation, facial recognition and emotional recognition. We’ve been working on developing that so that as well as a diagnosis we can provide additional tools to these kids.
CK: How could you hope to see AI being used across the medical industry going forward?
ST: AI is going to help us as clinicians become more efficient and give some of our time back to patients that we otherwise spend on tedious things.
The amount of time I waste on prior authorisation where I’m arguing with an insurance company to let me do something for a patient just shouldn’t happen. There’s an opportunity to use AI to give us back the joy of being a doctor.
There’s a lot of biases and disparities in healthcare, but I think that there is a way that AI actually helps to democratise medicine and allows people to have access to medicine that maybe they didn’t have before.
It’s a new tool, and a new tool sometimes is scary, and a new tool has to be monitored, but I think there’s a lot of hope for how AI can help us.