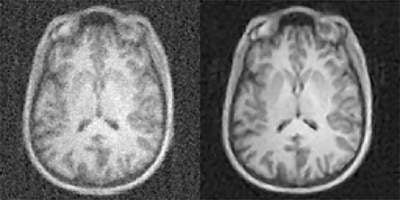
Researchers have developed a technique that uses artificial intelligence to obtain medical images without costly or dangerous increases in doses of radiation.
The team from the Athinoula A. Martinos Center for Biomedical Imaging at Massachusetts General Hospital called their technique AUTOMAP (automated transform by manifold approximation) in the paper ‘Image reconstruction by domain-transform manifold learning’ published in the journal Nature.
“An essential part of the clinical imaging pipeline is image reconstruction, which transforms the raw data coming off the scanner into images for radiologists to evaluate,” says Bo Zhu, research fellow at the Martinos Center and first author.
“The conventional approach to image reconstruction uses a chain of handcrafted signal processing modules that require expert manual parameter tuning and often are unable to handle imperfections of the raw data, such as noise.”
The AUTOMAP system is taught to ‘see’ in the same way that humans are taught to see; the team promoted the growth of neural connections through repeated training using real-world examples, rather than directly programming the software. AUTOMAP also takes advantage of recent developments in neural network models, graphical processing units and the availability of large stores of data, all of which are integral to the function of an image-based AI.
AUTOMAP also produces images quickly enough that doctors can make real-time decisions about patients while they are still being scanned.
How well do you really know your competitors?
Access the most comprehensive Company Profiles on the market, powered by GlobalData. Save hours of research. Gain competitive edge.
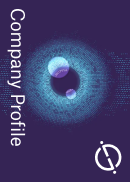
Thank you!
Your download email will arrive shortly
Not ready to buy yet? Download a free sample
We are confident about the unique quality of our Company Profiles. However, we want you to make the most beneficial decision for your business, so we offer a free sample that you can download by submitting the below form
By GlobalData“Our AI approach is showing remarkable improvements in accuracy and noise reduction and thus can advance a wide range of applications,” said Matt Rosen, senior author of the study, director of the Low-field MRI and Hyperpolarized Media Laboratory and co-director of the Center for Machine Learning at the Martinos Centre.
The technique could also aid in advancing other AI applications, many of which are based on clinical imaging and computer-aided diagnostics.
“We’re incredibly excited to have the opportunity to roll this out into the clinical space where AUTOMAP can work together with inexpensive GPU-accelerated computers to improve clinical imaging and outcomes,” said Rosen.