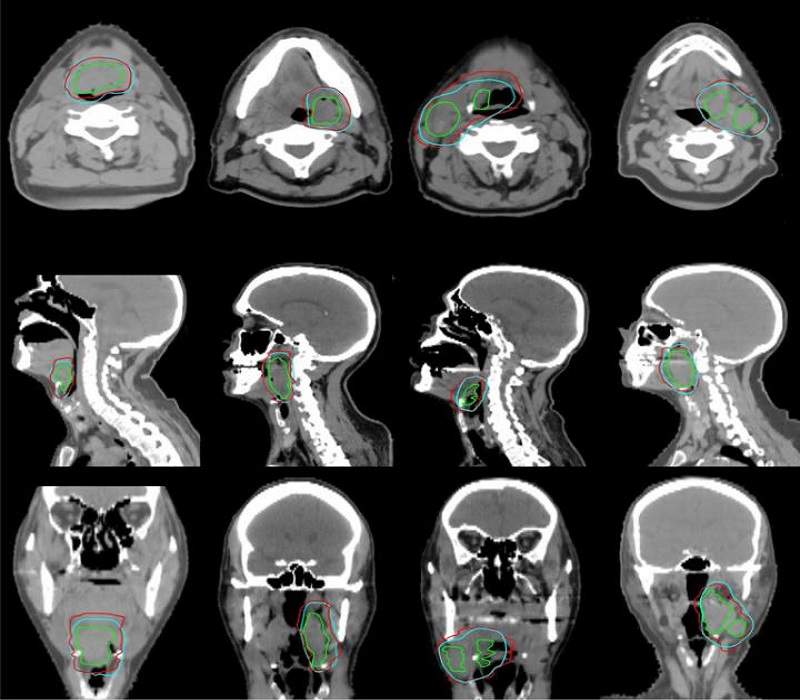
Researchers in the US have developed an artificially intelligent algorithm to accurately analyse tumours in cancer patients with a greater degree of speed and efficiency than physicians.
The algorithm, designed by scientists at the University of Texas at Austin, uses deep learning to identify gross tumour volume. This allows oncologists to determine how much radiation a patient will receive and how it will be delivered.
This process, known as contouring, has been found to show wide variability amongst physicians. A recent study from Utrecht University found this variability lead to some doctors suggesting high-risk clinical target volumes eight times larger than their colleagues.
“For high-risk target volumes, a lot of times radiation oncologists use the existing gross tumour disease and apply a non-uniform distance margin based on the shape of the tumour and its adjacent tissues,” said graduate research assistant Carlos Cardenas. “We started by investigating this first, using simple distance vectors.”
Cardenas and a team of researchers at MD Anderson analysed data from 52 oropharyngeal cancer patients who had been treated at MD Anderson between January 2006 and August 2010 and had previously had their gross tumour volumes and clinical tumour volumes contoured for their radiation therapy treatment.
“We have a lot of clinical data and radiation therapy treatment plan data at MD Anderson,” he said. “If we think about the problem in a smart way, we can replicate the patterns that our physicians are using to treat specific types of tumours.”
How well do you really know your competitors?
Access the most comprehensive Company Profiles on the market, powered by GlobalData. Save hours of research. Gain competitive edge.
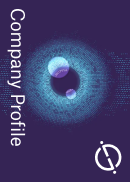
Thank you!
Your download email will arrive shortly
Not ready to buy yet? Download a free sample
We are confident about the unique quality of our Company Profiles. However, we want you to make the most beneficial decision for your business, so we offer a free sample that you can download by submitting the below form
By GlobalDataUsing this wealth of data Cardenas and his team developed a deep learning algorithm that uses auto-encoders, a form of neural networks that can learn how to represent datasets, to identify and recreate physician contouring patterns.
The paper describes using this process for the high-risk areas. When they tested the method on data that had been excluded from the training, they found the results were comparable to the work of a trained oncologist.
However, while an oncologist takes two to four hours to determine results, the machine learning method produces clinical target volumes in under a minute.
The Maverick supercomputer used in the study was provided by the Texas Advanced Computing Center (TACC), which sped up the lengthy process of training the system.
“If we were to do it on our local GPU, it would have taken two months,” said Cardenas. “But we were able to parallelise the process and do the optimisation on each patient by sending those paths to TACC and that’s where we found a lot of advantages by using the TACC system.”
Cardenas believes that integrating deep learning into cancer care has the potential to change the field, with some systems already making predictions with a higher accuracy than some radiologists.
“I hope that the clinical translation of these tools provides physicians with additional information that can lead to better patient treatments,” he said.
The results of the study will be published in the June 2018 issue of the International Journal of Radiation Oncology Biology Physics.