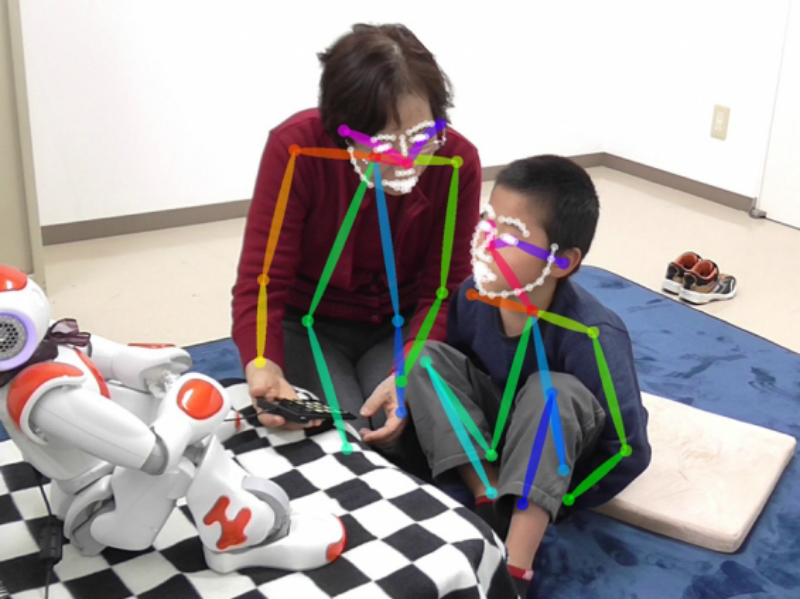
Researchers at the MIT Media Lab have developed a type of personalised machine learning that can help robots estimate the engagement and interest of autistic children during therapy sessions, using data that is unique to each individual patient.
The deep learning robots’ perception of the children’s responses agreed with assessments by human experts, with a correlation score of 60%. The scientists have published an associated report in Science Robotics.
The researchers used SoftBank Robotics NAO humanoid robots for the study. They are just under 2ft tall and resemble an armoured superhero. NAO can convey different emotions by changing the colour of its eyes, motion of limbs and voice tone.
The 35 children with autism who participated in this study ranged in age from three to 13. They reacted in a range of ways to the robots during their 35-minute therapy sessions, from looking bored and tired in some cases to excited, laughing or touching the robot in others.
MIT Media Lab postdoctoral researcher Oggi Rudovic said: “The long-term goal is not to create robots that will replace human therapists, but to augment them with key information that the therapists can use to personalise the therapy content and also make more engaging and naturalistic interactions between the robots and children with autism.”
The correlation scores of human observers reviewing an autistic child’s engagement are usually between 50% and 55%. Rudovic and his colleagues have suggested that trained robots like the one in their study could someday provide more consistent estimates of these behaviours.
How well do you really know your competitors?
Access the most comprehensive Company Profiles on the market, powered by GlobalData. Save hours of research. Gain competitive edge.
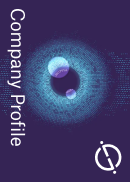
Thank you!
Your download email will arrive shortly
Not ready to buy yet? Download a free sample
We are confident about the unique quality of our Company Profiles. However, we want you to make the most beneficial decision for your business, so we offer a free sample that you can download by submitting the below form
By GlobalDataMIT researcher and co-author on the paper Rosalind Picard believes that personalisation is especially important in autism therapy because each case is different. She said: “The challenge of creating machine learning and AI that works in autism is particularly vexing because the usual AI methods require a lot of data that are similar for each category that is learned. In autism where heterogeneity reigns, the normal AI approaches fail.”
Rudovic and his colleagues built a personalised framework that could learn from data collected on each individual child. They captured video of each child’s facial expressions, head and body movements, poses and gestures, audio recordings and data on heart rate, body temperature, and skin sweat response from a monitor on the child’s wrist.
The robots’ personalised deep learning networks were built from layers of these videos, audio and physiological data, information about the child’s autism diagnosis and abilities, their culture and their gender. The researchers then compared their estimates of the children’s behaviour with estimates from five human experts, who coded the children’s video and audio recordings on a continuous scale to determine how pleased or upset, how interested, and how engaged the child seemed during the session.