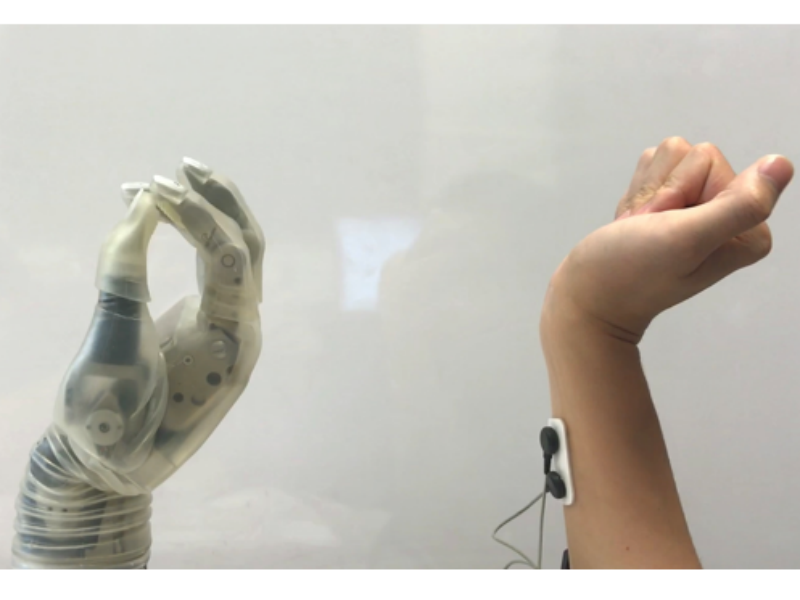
Researchers in the joint biomedical engineering program at North Carolina State University and the University of North Carolina at Chapel Hill have developed a technology which can decode neuromuscular signals to control powered prosthetic wrists and hands.
The technology relies on computer models that closely mimic the behaviour of the natural structures in the forearm, wrist and hand and could make prosthetics easier to use for patients.
Currently available powered prosthetics use machine learning to create a ‘pattern recognition’ approach to prosthesis control but this technique has several flaws that the researchers wanted to address.
Senior author of the study Professor Helen Huang said: “Pattern recognition control requires patients to go through a lengthy process of training their prosthesis. This process can be both tedious and time-consuming.
“We wanted to focus on what we already know about the human body. This is not only more intuitive for users, it is also more reliable and practical.
“That’s because every time you change your posture, your neuromuscular signals for generating the same hand/wrist motion change. So relying solely on machine learning means teaching the device to do the same thing multiple times; once for each different posture, once for when you are sweaty versus when you are not, and so on. Our approach bypasses most of that.”
How well do you really know your competitors?
Access the most comprehensive Company Profiles on the market, powered by GlobalData. Save hours of research. Gain competitive edge.
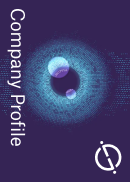
Thank you!
Your download email will arrive shortly
Not ready to buy yet? Download a free sample
We are confident about the unique quality of our Company Profiles. However, we want you to make the most beneficial decision for your business, so we offer a free sample that you can download by submitting the below form
By GlobalDataThe researchers developed a user-generic, musculoskeletal model as an alternative to pattern recognition. To do this they put electromyography sensors on the forearms of six able-bodied volunteers and tracked which neuromuscular signals were sent where as the volunteers performed different actions with their wrists and hands. They then used this to create a generic model, which translated those neuromuscular signals into commands that can manipulate the prosthetic.
Huang said: “When someone loses a hand, their brain is networked as if the hand is still there. So, if someone wants to pick up a glass of water, the brain still sends those signals to the forearm. We use sensors to pick up those signals and then convey that data to a computer, where it is fed into a virtual musculoskeletal model.
“The model takes the place of the muscles, joints and bones, calculating the movements that would take place if the hand and wrist were still whole. It then conveys that data to the prosthetic wrist and hand, which perform the relevant movements in a coordinated way and in real time – more closely resembling fluid, natural motion.”
Both able-bodied and amputee volunteers were able to use the model-controlled interface to perform all of the required tasks despite having very little training. The researchers are now looking for volunteers with transradial amputations so they can gather further feedback.
The technology has proven it can work well during early testing but has not yet entered clinical trials, which means it is years away from commercial availability.